Our work at diversitydatakids.org is aimed at finding new and better ways of using data to improve children’s lives and increase racial/ethnic equity. Inequities are already manifest at birth and result in drastically unequal survival chances for infants born to Black and White mothers: 1 in 93 Black infants dies before their first birthday, but only 1 in 217 White infants dies. However, the standardized collection of data around the time of birth also constitutes an opportunity for addressing these inequities through better use of data. At the time of birth, detailed socio-demographic and health data is collected for all mothers and their newborns in the U.S.—through the standardized U.S. birth certificate. Can this data be leveraged to accurately predict adverse developmental outcomes at the time of birth? And could risk scores derived from such predictions inform interventions aimed at improving survival chances and developmental outcomes for those infants most at risk? What would the implications of a use of algorithms be for racial/ethnic inequality? Our research points to promising approaches to reduce existing racial inequities in birth outcomes.
In a new study lead by Antonia Saravanou, a PhD student in computer science at the University of Athens, we take a machine learning approach to predict, at birth, newborn infants’ risk of dying within the first year of life. To our knowledge, this is the first study attempting this task using birth certificate data for all U.S. births. We use publicly available data for all children born in 2000 and 2001 to train machine learning algorithms, and test the algorithms predictions using data on all births in 2002.
Our best performing models achieve high accuracy: 77% of infants that the model identifies, at birth, to be at the highest risk of dying do not survive until their first birthday. However, many of the infants predicted to die, in fact, survive. This is to be expected because infants with many characteristics that put them at risk of dying, for example, being born very preterm, also receive extensive medical care after birth, which increases the odds of survival substantially. We find that the algorithms perform better in predicting deaths that occur soon after birth and deaths from specific causes, such as those related to very preterm birth. We also find that the models are slightly more accurate for infants born to Black mothers compared to infants born to White mothers.
One limitation of our finding is that the risk scores are obtained in relation to a specific outcome, i.e., survival until the first birthday. However, other research has shown that the factors predicting infant survival also predict, for example, neurodevelopmental impairment or delayed and cognitive development. More importantly, to date, very little research is available on the practical feasibility of using risk scores to inform practice. The algorithms permit the calculation of risk scores at birth for every infant born in the U.S. And subsequent care provision or program enrolment both within and beyond the clinic could be informed by these risk scores. However, practical and ethical implications of using risk scores in this way still need to be worked out.
Nevertheless, from an equity perspective, our study has several strengths and our initial results point in the right direction: The algorithms are trained on data for all births occurring in the U.S., which makes it less likely that risk scores obtained from them are distorted by selective data collection or sampling. The risk scores reflect that infants born to Black mothers tend to have poorer birth outcomes and are therefore at higher risk of dying; the algorithm therefore assigns higher risk scores to infants born to Black mothers. The algorithms are also slightly more accurate for babies born to Black mothers. These are desirable properties for anti-racist policies or interventions informed by algorithmic risk scores. For example, if an intervention boosting survival is equally effective for White and Black infants, allocating infants to this intervention based on the risk score should in tendency increase equity in survival rates. Building on this work, we are currently examining potential practical applications. We hope that this research can contribute to ongoing efforts to shape anti-racist health policies aimed at reducing the unacceptably high rates of infant mortality for Black infants in the U.S.
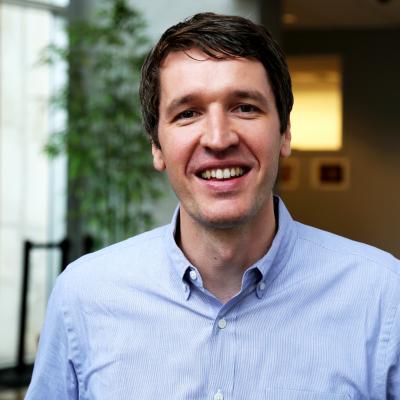
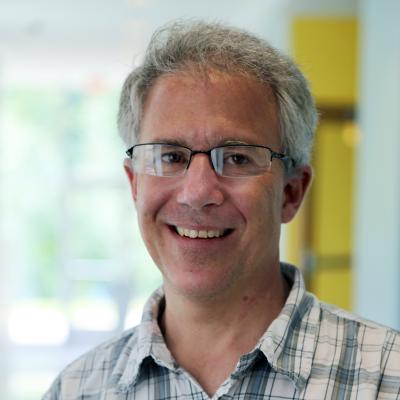
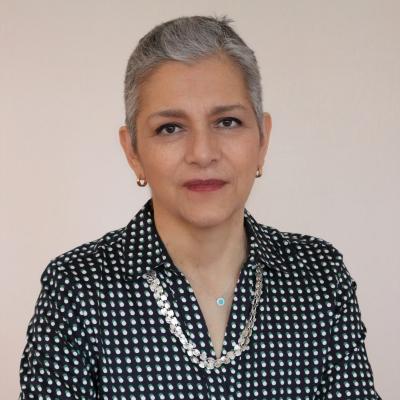